Bias-corrected CMIP6 global dataset improves dynamical downscaling projection of future climate
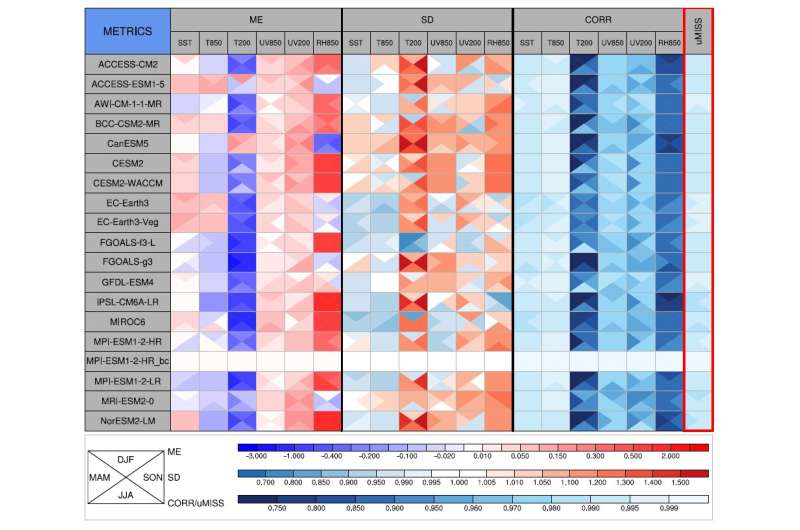
Projections of the Earth's future climate at a finer scale are important in climate-related studies. However, the typical spatial resolution of CMIP6 models is approximately 100 km, which is not sufficient for resolving fine-scale orography, land cover and dynamics of the atmosphere, hindering their ability to simulate extreme weather and climate events.
Dynamical downscaling method with a regional climate model is an important approach to obtaining fine-scale weather and climate information; whereas the traditional dynamical downscaling simulations are often degraded by biases in the global climate model (GCM).
Recently, a new study published in Scientific Data reported a novel GCM bias correction method, which takes advantage of the non-linear long-term trend of ensemble mean of 18 CMIP6 models to reduce the uncertainty of future projection generated by a single GCM. Moreover, both the GCM mean and variance biases were corrected based on the ERA5 reanalysis data.
Using this GCM bias correction method, the researchers developed a set of bias-corrected large-scale forcing data with a grid spacing of 1.25 longitude by 1.25 latitude based on the ERA5 reanalysis and CMIP6 data. The bias-corrected dataset included three surface variables and eight upper air variables for three sets of bias-corrected CMIP6 data, the historical data from 1979 to 2014, and SSP245 and SSP585 from 2015 to 2100.
"The bias-corrected GCM data shows much better quality than individual CMIP6 models and can provide high-quality large-scale forcing for dynamical downscaling projections of the Earth's future climate, atmospheric environment, hydrology, agriculture, wind power, etc.," said Dr. Xu Zhongfeng from the Institute of Atmospheric Âé¶¹ÒùÔºics (IAP) of the Chinese Academy of Sciences, the first author of the study.
More information: Zhongfeng Xu et al, Bias-corrected CMIP6 global dataset for dynamical downscaling of the historical and future climate (1979–2100), Scientific Data (2021).
The dataset is accessible at:
Provided by Chinese Academy of Sciences